As part of the NWO SSHOC-NL research infrastructure project (2024-2028), the Platform for Large-scale Analysis of NETworks (PLANET-NL) aims to develop tools and algorithms for analyzing large scale datasets with (digital) traces of socio-economic interaction. We develop disclosure risk-aware methods and tools specifically in the context of combining different social network data sources (micro data registers, demographic databases, social media, etc.) at different levels of, e.g., social and geographical aggregation. For more information about PLANET-NL, see the PLANET-NL website.
bookmark_borderNew project: PLANET-NL – Platform for Analysis of Large-Scale NETworks
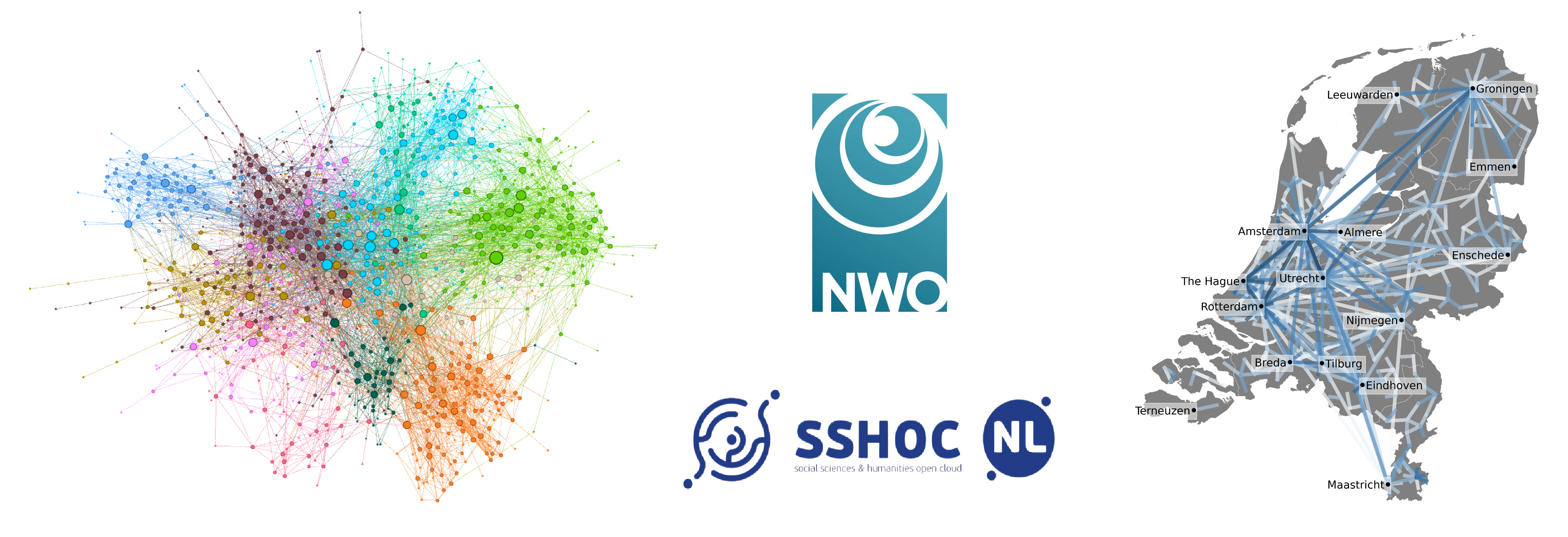